IIT Delhi Develops ML, Cloud Computing Based Tool For Automated Mapping Of Landslide Extent
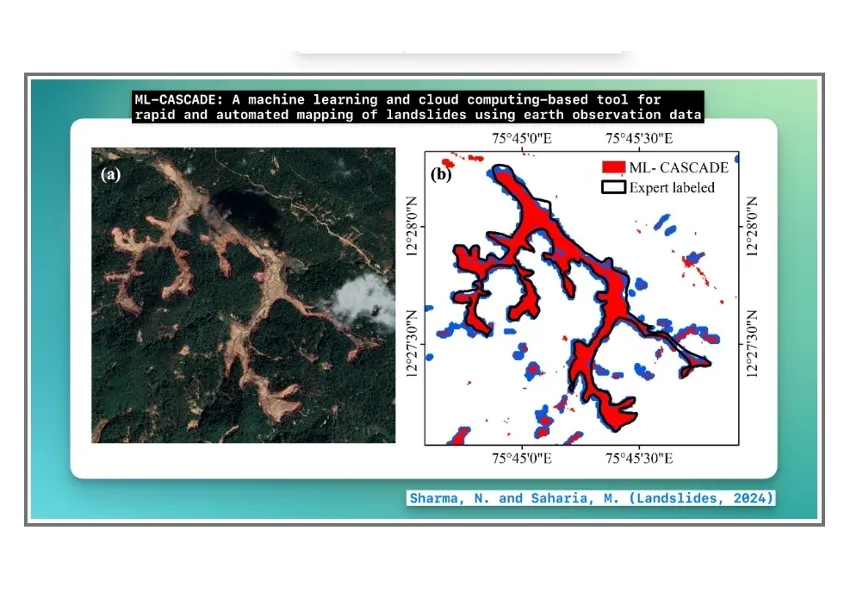
Researchers from the Civil Engineering Department at IIT Delhi have developed a cloud computing and machine learning-based tool, ML-CASCADE, to map landslide extent using satellite data.
This publicly-available tool requires only two inputs, i.e., an approximate date and location of a landslide event and accurately maps a complex cluster of landslides within 5 minutes and a simple landslide within 2 minutes, which is critical for post-disaster damage assessment. The underlying model is trained on a large amount of satellite, terrain, vegetation and soil data.
A research paper by PhD Scholar Nirdesh Kumar Sharma and Prof Manabendra Saharia of the HydroSense Lab at the Civil Engineering Department has been published in the ‘Landslides’ journal by the International Consortium on Landslides (ICL).
The landslide problem is treated as a binary image segmentation problem. Nineteen features have been used to develop the model with Sentinel-2 bands (pre- and post-landslide), slope and aspect from NASA Digital Elevation Model data, Normalised Difference Vegetation Index (NDVI) and differential Bare Soil Index, which can detect fresh landslide development.
The decision-tree-based classification model allows both pixel and object-based methods for segmentation. The novelty of this tool is that it is a dynamic machine learning model that does not depend on pre-trained models that require large annotated training data unavailable for landslides as well as limited transferability to new areas. Instead, it builds a custom model based on user-provided inputs and satellite imagery, adapting to local terrains and specific environmental factors.
To provide a simple and practical tool to disaster managers, the user interface has been designed in consultation with both technical and non-technical users. The final output is easily downloadable in multiple image formats, which can be disseminated or used for further processing in GIS. With Google Earth Engine as a backend, it requires no downloading of data and using parallel processing, the outputs take only minutes to generate, which would take hours on a local computer.
The tool has been extensively validated over thousands of landslides, with two major events in Himalayas (Kotrupi landslide, 2017) and Western Ghats (Kodagu landslide, 2018) provided as case studies in the paper. Most landslides remain unmapped since they happen in far off locations and developing a comprehensive inventory requires significant domain expertise, time and effort. This tool is now being used by IIT Delhi researchers to develop a national historical landslide inventory with spatial extents, which will be invaluable in developing landslide early warning systems.
The architecture of the tool has future potential applications in mapping flood inundation, deforestation, sand mining and other environmental challenges.